Future of AI Text Detection: Challenges in Identifying AI-Generated Content
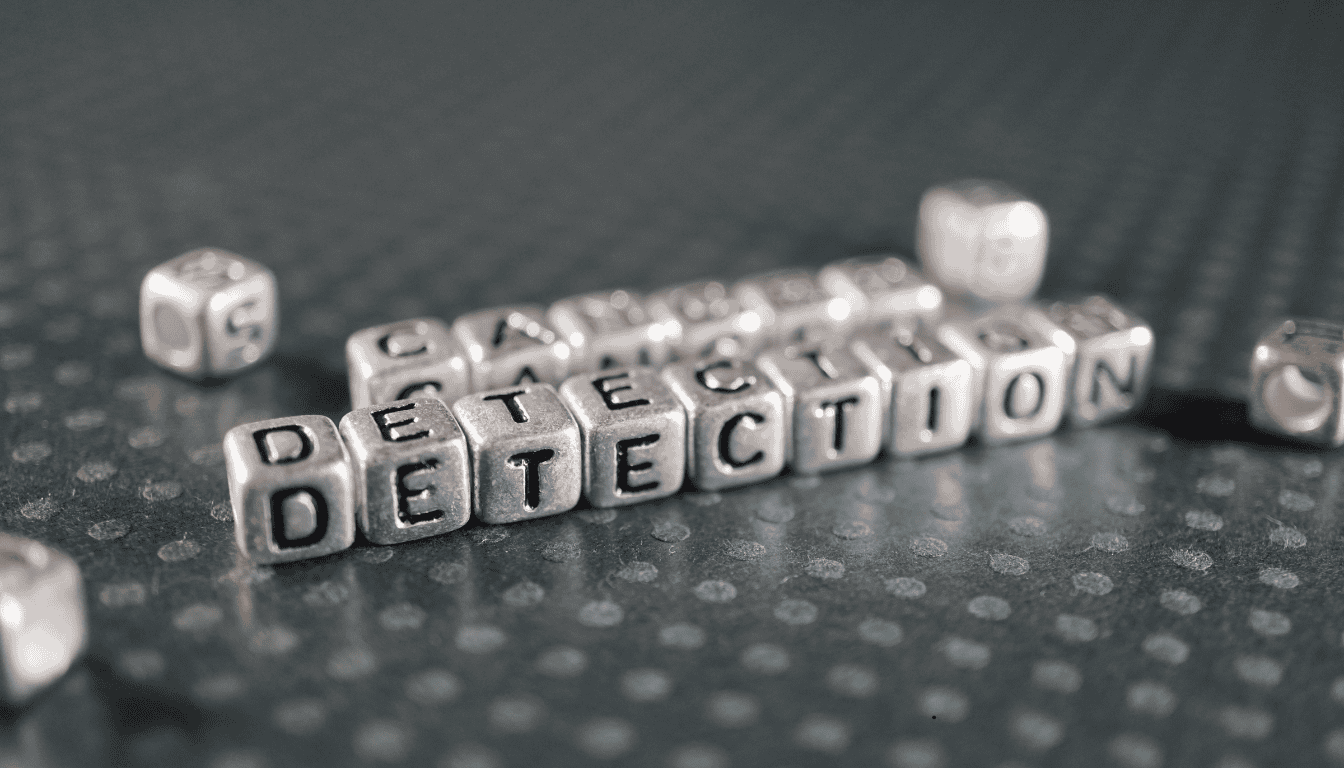
A 2023 study showed that AI detection tools can’t tell AI-written from human text well. This highlights the evolving nature and challenges of ai content detection in distinguishing between human and AI-generated content, showing we need better natural language processing for AI text detection in the future.
AI content is getting smarter, making it harder to spot. Current ai content detection tools can’t keep up with new language models. This shows we must create strong detection methods to keep content true and real.
The world of AI text detection is changing fast. OpenAI leads with 36% of the market and is worth over 80 billion USD in 2024. But even leaders like OpenAI have problems. They stopped their ai detection software because it wasn’t reliable in schools.
The future of AI text detection is bright with better natural language processing and deep learning. These new techs will help us better understand AI-generated content. This could change how we check content for realness and truth, addressing the benefits and challenges associated with artificial intelligence in content detection.
AI Generated Content
I’ve seen a big shift in how we create content. AI tools are being used everywhere. They write news, answer customer questions and even create stories. This is making us rethink who or what is behind the content we see every day.
Digital Marketing and Content Creation
AI writing tools are changing digital marketing. They can write fast, an article in minutes. Many of these tools cost $100 a month for a lot of words. They help with SEO by suggesting keywords and creating content that search engines like. But Google is now looking closely at content made just for rankings, using content detection tools to identify AI-generated content and ensure content integrity.
Challenges with Advanced Language Models
While AI tools are fast, they lack human touch. They can’t be truly creative or personal. This limits how well they can engage readers. There’s also copycat concerns. AI learns from existing text which can lead to content that’s too similar to other sources. Optical character recognition and deep learning for text helps AI read and understand but can’t replace human insight. However, detecting AI-generated text remains challenging due to the limitations of current methods, such as pattern recognition and watermarking, which struggle to keep up with the evolving nature of AI writing technologies.
We Need Better Detection Methods
As AI content grows, detecting AI-generated content becomes crucial due to the growing need for reliable detection methods. This matters for trust in news, schools, and online info. OpenAI is working on tools to detect AI text. These use advanced text analytics to find machine-made content. In the end, even with AI help, we still need humans to check and improve what AI writes.
Current State of AI Text Detection
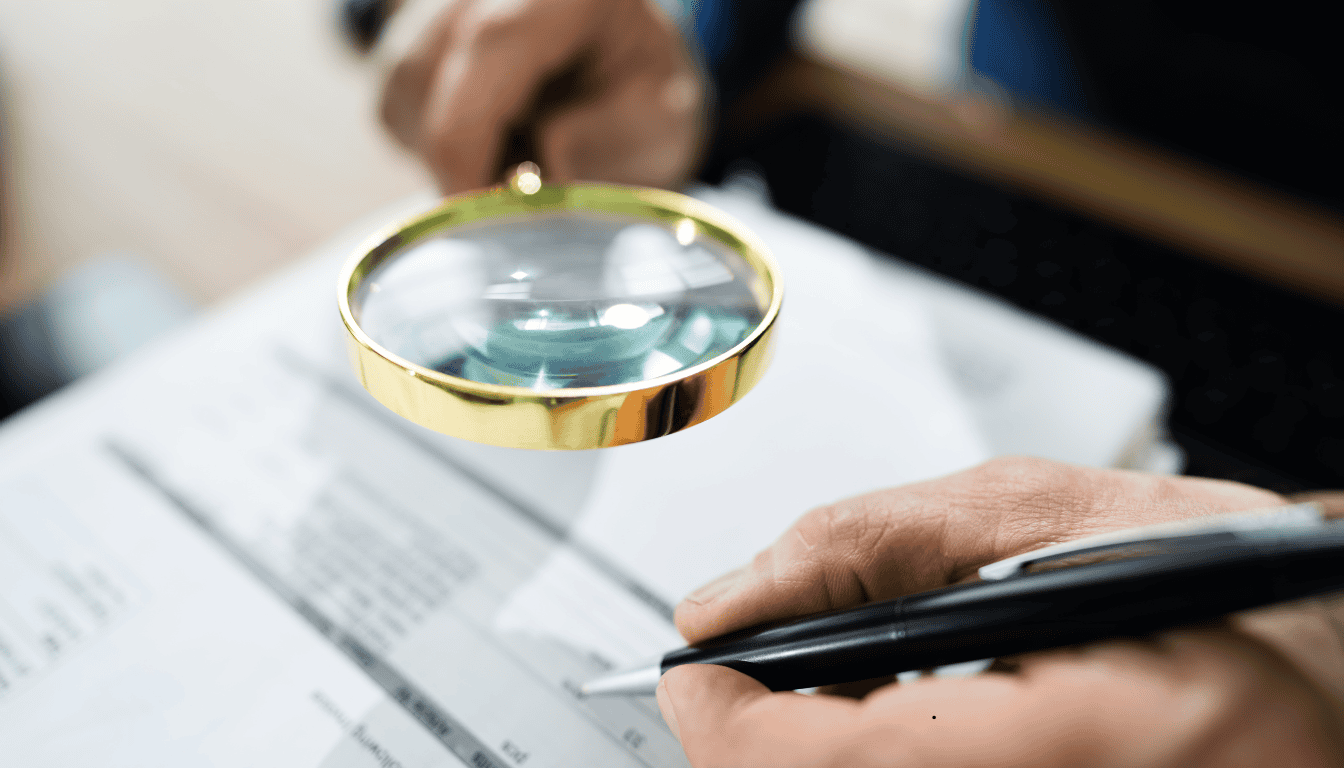
I’ve been looking into the latest in AI text detection, and it’s really interesting. Now, computer vision and text extraction help find AI-made content. The best AI systems can spot AI-written text with up to 95% accuracy. This is way better than humans, who can only do about 53%.
AI text mining is now key because tools like ChatGPT and Google Gemini make content that sounds very human. A study found 3.3% of student papers had over 80% AI-written text. This shows how hard it’s getting for teachers to identify AI generated content. Now, 43% of teachers worry most about stopping cheating, up from just 15% before.
Even with these advances, AI text detection has its challenges. For example, Turnitin missed 10 out of 16 AI-written papers in a test recently. This shows we need more research and development. As AI language models get better, we must improve detection methods to accurately identify AI generated text. This ensures the integrity of written work in all areas.
NLP Progress
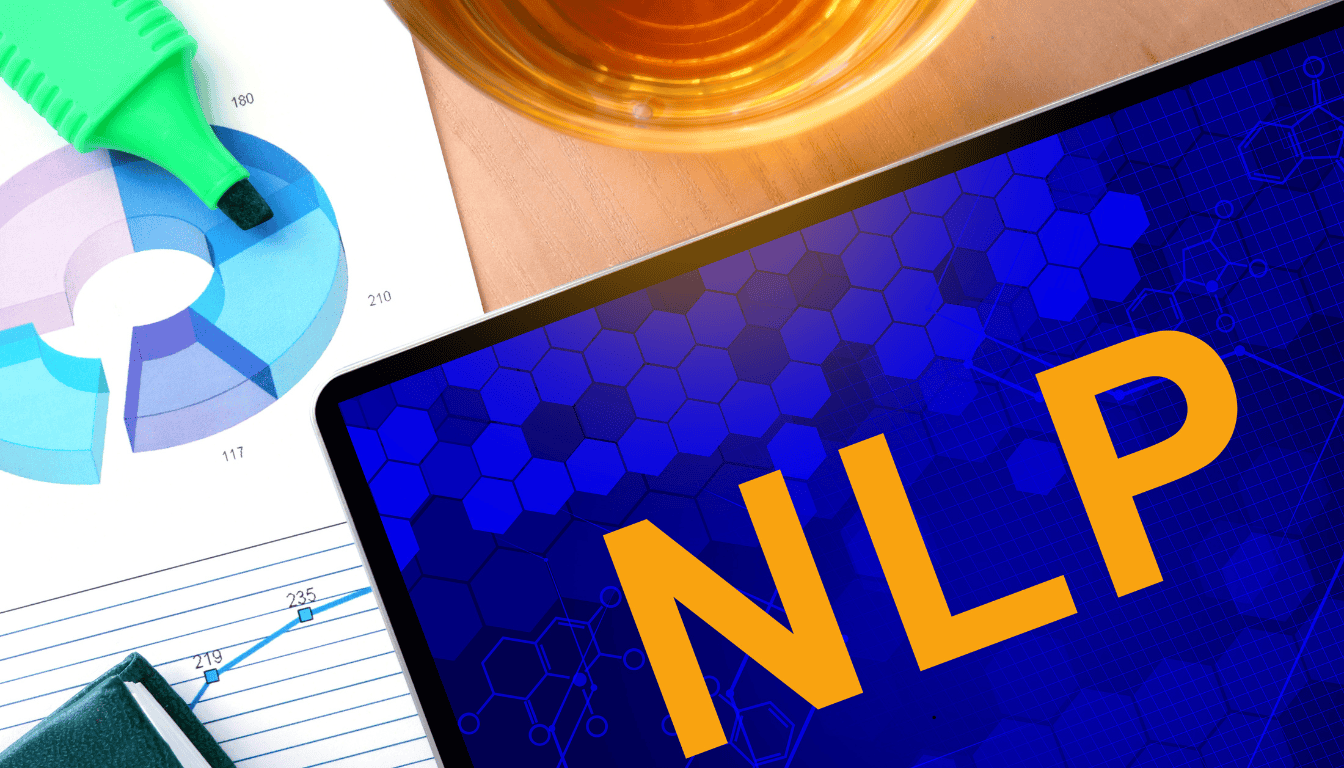
NLP has come a long way recently, largely due to advancements in machine learning. I’ve seen how language models, powered by machine learning, change the way we talk to machines. It’s moving fast, new things are happening all the time.
Generative AI is also making a significant impact across multiple sectors, including journalism, drug design, and industrial design. Research reports outline both the opportunities and challenges presented by this technology, with case studies from companies successfully integrating generative AI into their operations.
Deep Learning for Text
Deep learning for text has changed the way we analyze language. Neural networks now understand context and subtlety better than before. This has improved translation, summarization, answering questions, and detecting AI-generated text.
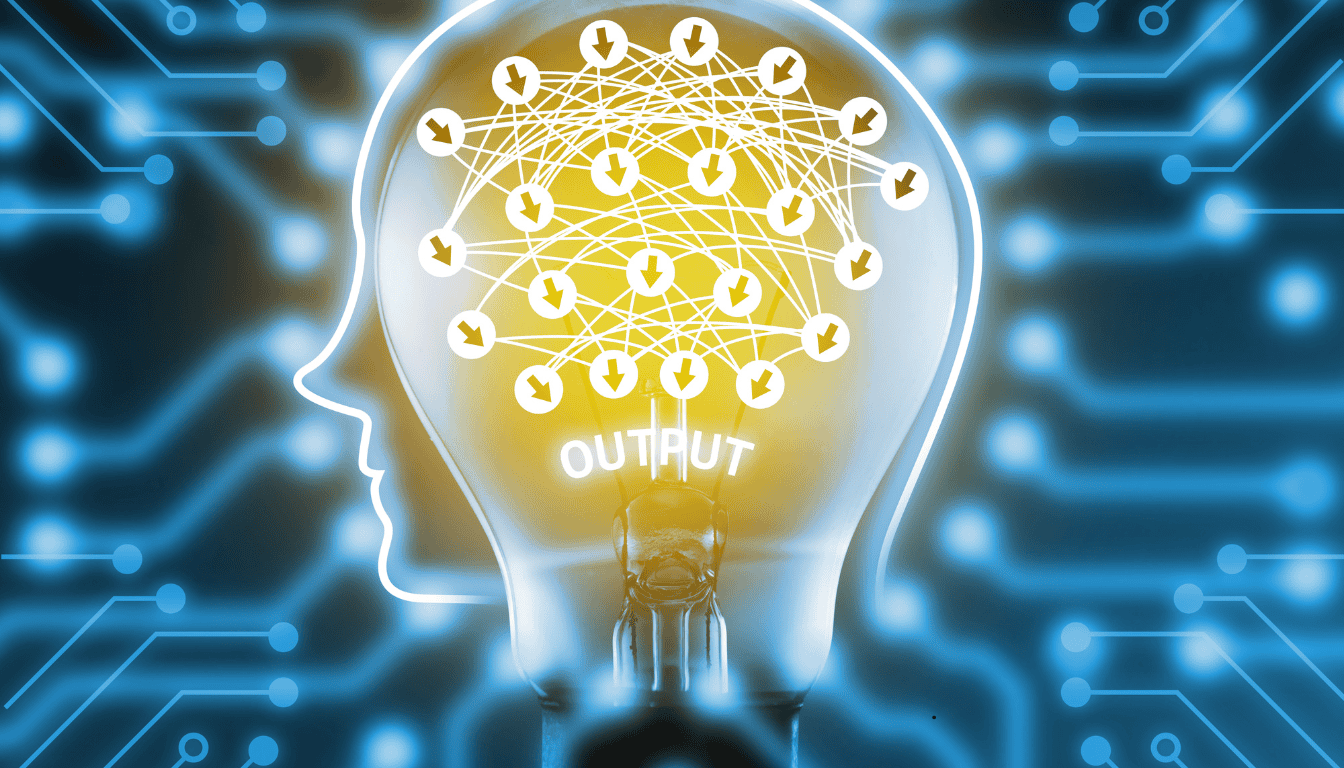
Transformer Models and Their Impact
Transformer models have changed NLP. These models can process text fast and accurately. They help chatbots and virtual assistants talk more naturally to humans.
Semantic Meaning Improvements
We’ve made a lot of progress in semantic understanding. Today’s language models understand meaning and context much better. This means better text analysis and generation. It’s cool to see this happening in healthcare, finance and customer service.
The future of NLP looks good. As deep learning gets better we’ll see more cool things. It’s a great time to be in this space.
The future of AI text detection is cool but tricky. Studies show current detectors can fail in real life. For example, one detector thought the U.S. Constitution was AI written! We need better tools to analyze text with AI.
Emerging Tech and Techniques
Researchers are finding ways to make text analysis better with AI detection software. They’re looking at bigger chunks of text, like paragraphs or documents. This gives AI more to work with. I think future detectors will use smart semantic analysis and intent detection to detect different types of content better.
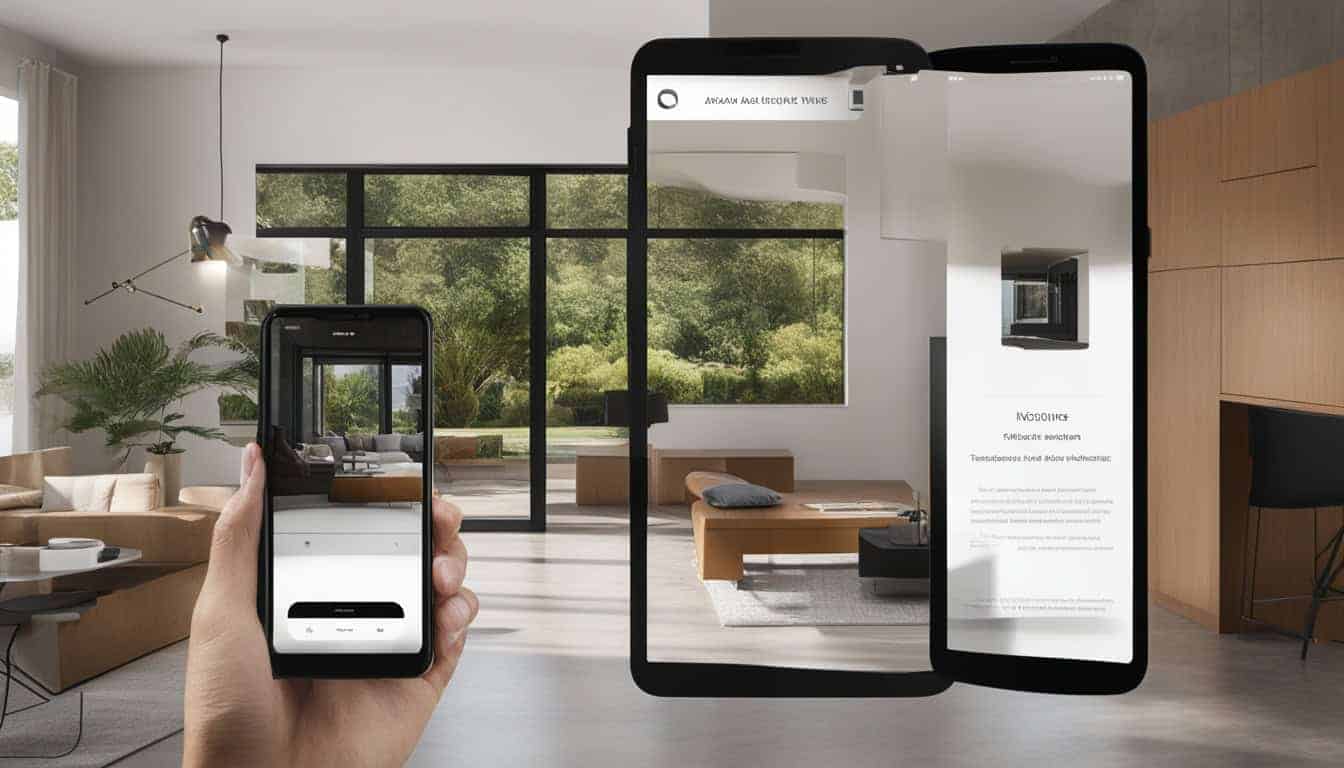
Multi-Modal Detection
The future of AI text detection will include AI content detection tools that analyze text, images, videos, and audio. So we can get a better understanding of content. That’s a big deal that could change how we detect AI generated content.
Real-Time Detection
Real-time detection is a big deal. Future AI tools might give us instant feedback and tips. That would make content creation faster and better. Systems will learn from us, get smarter over time.
Now that AI text is everywhere, we need to talk about this. We need to create rules and better detection. We’ll use AI smartly and not misused.
Ethical Considerations in AI Text Detection
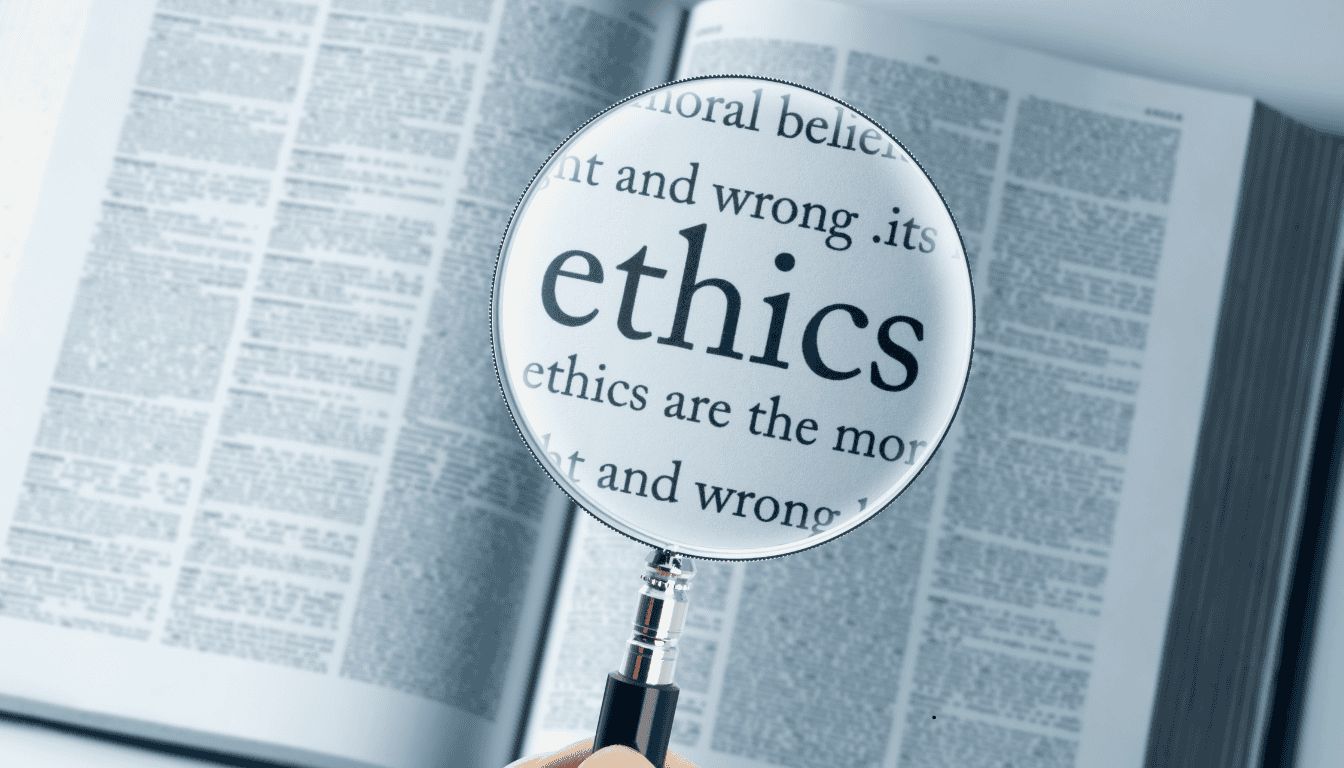
I’ve been thinking about the ethical sides of AI text detection. As AI gets better at understanding language, we face new issues. The White House’s $140 million for AI shows how important this tech is. But we must use it wisely.
AI text mining can be a threat to privacy. It looks at a lot of data, which could risk our personal info. We need to find a balance between new tech and protecting our rights. The ban on ChatGPT in some US schools shows how worried we are about cheating.
AI can also show bias if we’re not careful. It’s only as fair as the data it learns from. If we’re not careful, AI could keep old prejudices alive. U.S. agencies warn us about the need for fair AI.
AI can spread false info too. As AI gets better at writing, it’s hard to tell real from fake text. This could harm our society and democracy a lot.
As we move forward, using AI wisely is key. We should see AI as a tool to help us, not replace our creativity and thinking.
AI Text Detection in Academia and Research
I’ve noticed a big need for AI text detection in schools. As AI gets better, keeping things honest is key. We need to tell AI-made content from human generated text. Tools like optical character recognition and text extraction help with this.
Preserving Academic Integrity
AI text analysis is key for maintaining academic integrity by detecting AI-generated text. Studies show that AI detection tools are not all the same. Originality.ai can spot AI-made texts every time, and ZeroGPT catches 96% of ChatGPT texts. These tools help catch plagiarism and keep research quality high.
Tools for Peer Review and Verification
New tools, including AI detection software, are coming out to help with checking each other’s work in peer review. Turnitin finds 30% of AI-changed articles but doesn’t mistake human work. These tools use special text extraction to look at writing style. They’re becoming a big part of how research is checked.
Impact on Scientific Publishing
AI-generated content is changing how research is shared. Journals are using AI detection tools in reviews. This keeps published research trustworthy. As AI text analysis gets better, it will be a key part of sharing research, keeping the integrity of science high.
The Role of AI Text Detection in Combating Misinformation
I’ve noticed a lot more AI-generated content lately. That’s why ai text mining is so important. It helps us find the truth in all the noise.
Text analytics is key in this fight. It spots writing patterns we might overlook. For instance, AI content often lacks depth or has strange phrasing. By noticing these things, we can quickly spot fake news.
The future of ai text detection is thrilling. New tools are getting better at finding AI-made content. They check for sentence variety and if ideas flow smoothly. This makes our online world more honest.
But, these tools aren’t perfect yet. They can wrongly flag human writing as AI or miss AI text. That’s why we must keep improving them. Our aim is to have systems that can quickly and accurately catch fake news by being able to identify AI-generated text.
As we approach big elections in 2024, this technology will be vital. It’ll help us fight false info on social media. By using smart AI to detect fake AI, we’re working towards a more trustworthy internet for all.
AI Text Detection Challenges
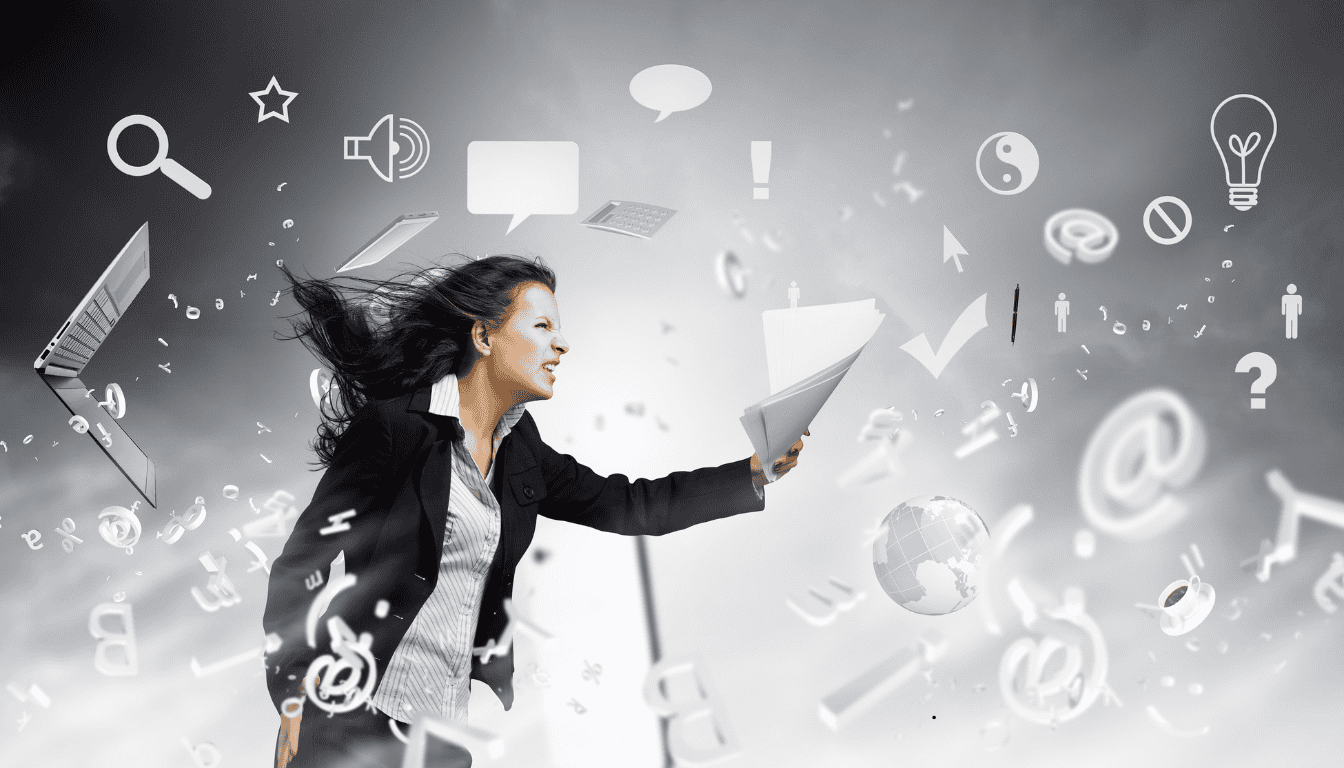
Building AI text detection systems is hard. As the challenge to detect AI-generated text grows, language models change fast and developers have to keep up with the AI content.
Language Models and Detection Evasion
Deep learning for text makes it hard to tell human from machine written content. Some detectors say they’re 99% accurate. But they fail with texts from other models, highlighting the limitations of AI detection software in accurately identifying content created by different artificial intelligence models.
Accuracy vs False Positives
Finding the balance is tough. Some systems flag human content as AI written up to 70% of the time. That hurts trust and targets human writers unfairly.
Scale and Computational Resources
Handling big data is another big challenge. The RAID dataset has over 10 million documents. It requires a lot of computing power. As AI models get more complex so do the requirements of detection systems. We need to make efficient algorithms that can handle large amounts of data.
Joint Efforts in AI Text Detection Research
I’ve been looking into the future of AI content detection. Researchers from different fields are teaming up to solve the hard problems of detecting AI written content. That’s key as AI gets better at text analysis.
The CoAuthor dataset is a big deal. It has texts written by humans and AI together. This dataset helps researchers get better at detecting AI written parts in human written and AI written texts. Nice to see AI being used to detect AI in these mixed texts.
Now countries are working together more. For example, six European projects are collaborating for four years to build AI tools that detect fake content. They’re not just looking at text. They’re also looking at deepfakes and doctored images. That could be a game changer in the fight against fake news.
We need to share data and methods to move forward in AI text detection. The field is moving fast. Let’s work together to keep up. Nice times ahead!