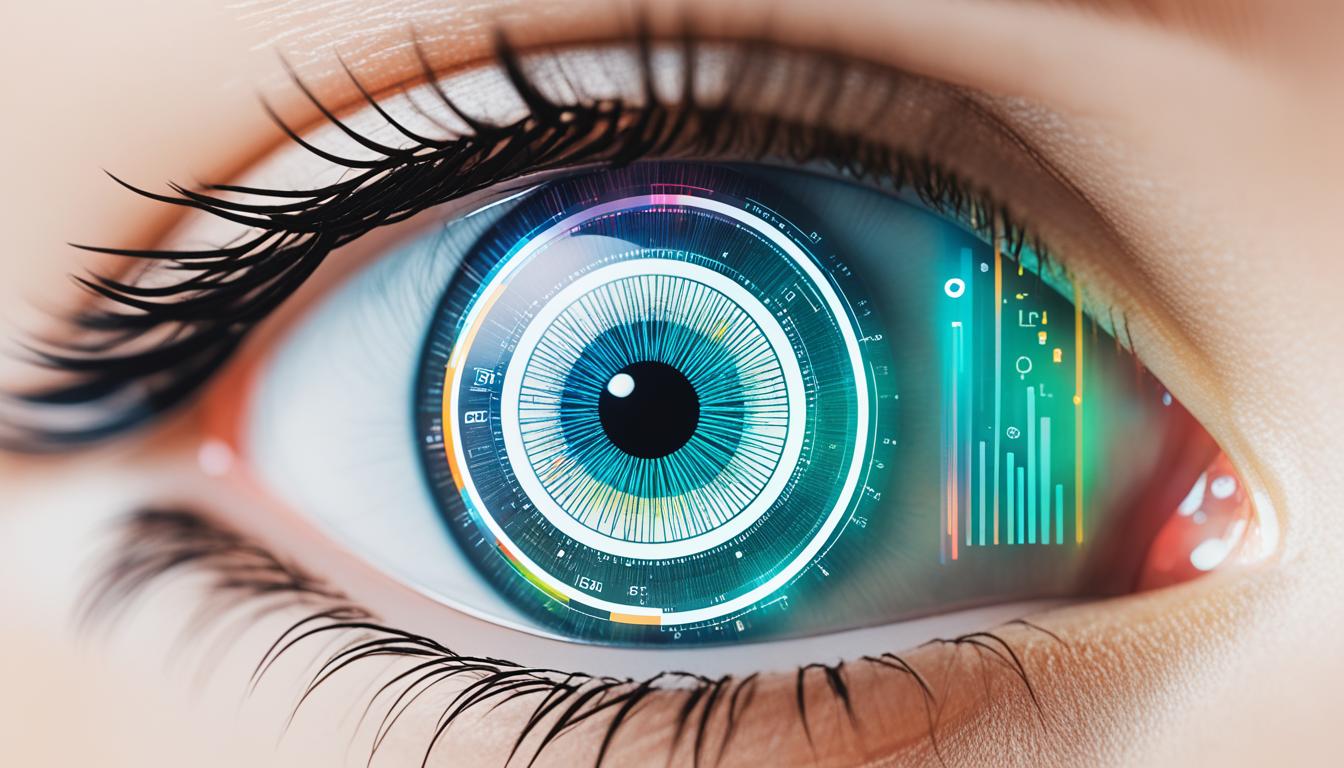
Did you know AI content detectors are right 7 out of 10 times in 100 articles? This fact shows how important an AI detector is today, as these tools are specifically designed to analyze and assess the authenticity of written content. As more content is made by AI, knowing how these systems work is key for creators and users.
Iโm excited to share the world of AI text detection with you. Weโll look at how machine learning and natural language processing work in these tools. Weโll see the main technologies that help AI detect content.
But, AI detection isnโt perfect. Between 10% and 28% of articles thought to be human-written were actually AI-made in a test of 100 articles. This shows the challenges AI systems have in telling human and machine-made text apart.
Weโll dive deeper into AI detectorsโ accuracy and limits, their uses, and the debate on their trustworthiness. This is for content creators, marketers, or anyone curious about AI technology. Youโll learn a lot about AI text detection in this journey.
Key Takeaways
AI content detectors are about 70% reliable in identifying AI-generated text
False positives occur in 10-28% of human-written content
Machine learning and natural language processing are key technologies in AI detection
AI detectors analyze features like classifiers, embeddings, perplexity, and burstiness
Manual review is often necessary to ensure accuracy in AI text detection
Introduction
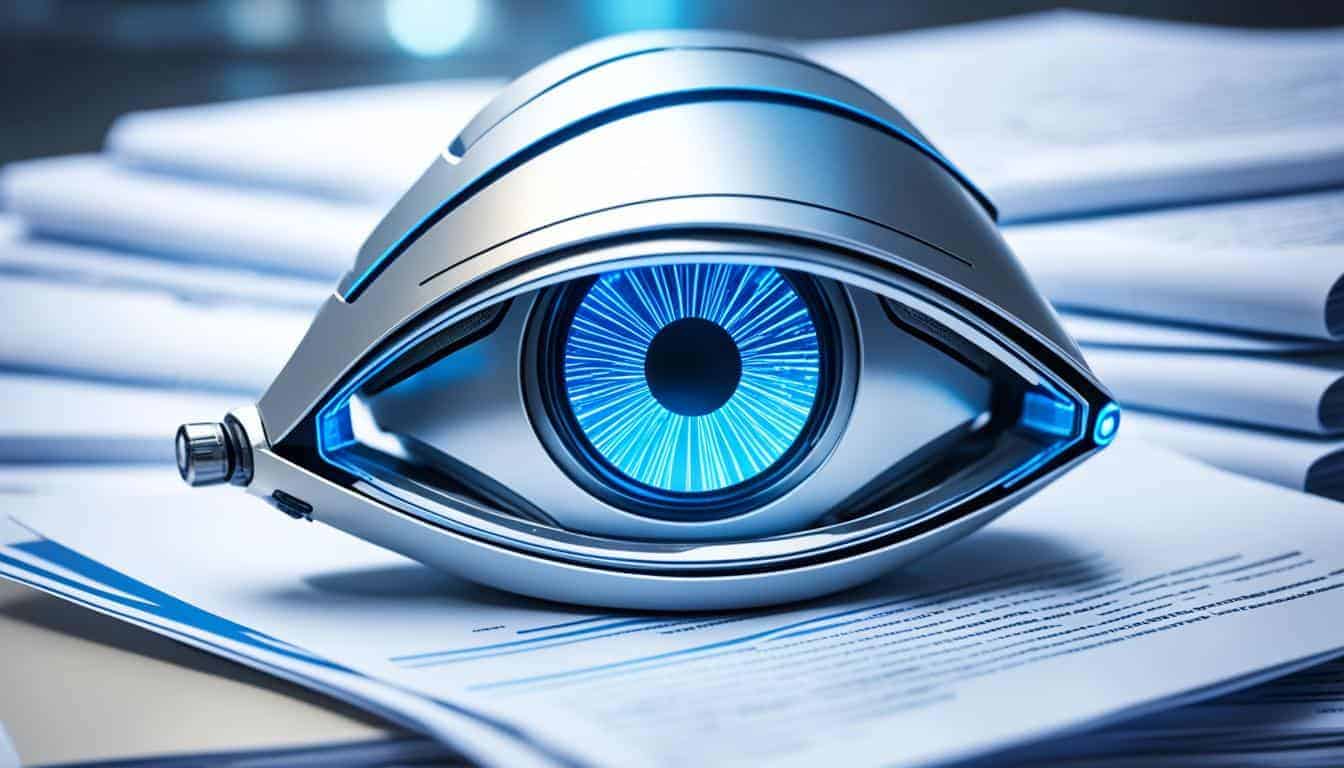
Iโve noticed more people using artificial intelligence for making content. This has made us need an AI detection tool to spot AI-written text through algorithmic analysis. As a writer, Iโm really interested in how these tools work and their effect on content.
Tools for finding AI content use complex algorithms to check text. They look for patterns that suggest AI was used. These systems use text analysis to find signs of machine writing. They aim to tell apart human and AI-made content. Agencies, content creators, and educational institutions often rely on an AI detector to ensure content integrity and combat academic dishonesty.
These detectors arenโt always right. In a test, over 20% of texts written by humans were marked as AI-made. This shows the challenges in finding AI content. It tells us these tools are still getting better.
Google doesnโt mind if content is AI-written. They focus on quality, not how itโs made. This lets writers use an AI writing tool. The important thing is to use AI smartly, mixing it with human creativity for great content.
What is AI Content Detection?
AI content detection is a new tech that checks if text was made by a machine or a person. It uses advanced analysis to look at writing patterns. Computational linguistics is also part of these advanced techniques, helping to understand and analyze language use. An AI language model generates patterns that can be recognized by an AI detector, an AI tool specifically designed to analyze and assess the authenticity of written content. These detectors help identify machine-generated content by comparing the text to lots of human and AI texts in real-time.
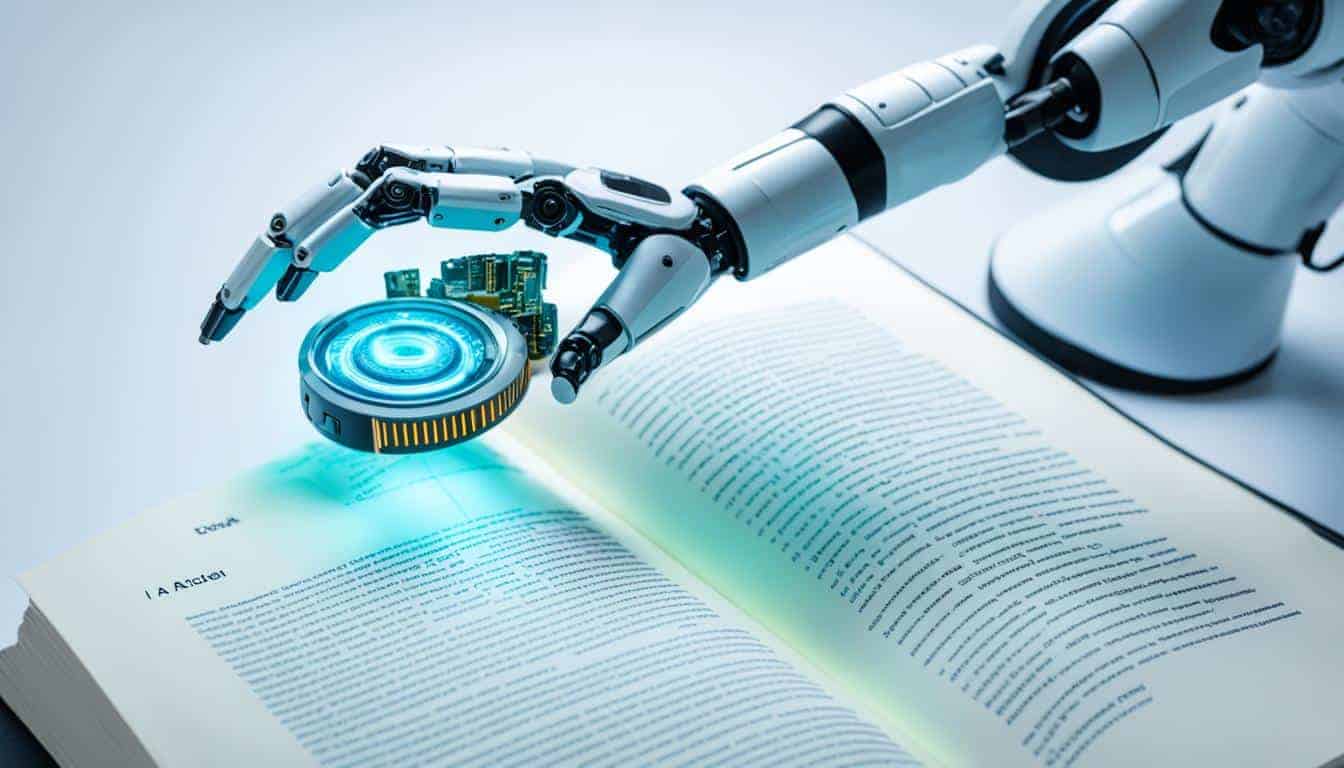
This tech looks at the meaning, structure, and language used in texts. It checks things like perplexity and burstiness. Perplexity is how well a model predicts a text. Burstiness is about the mix of short and long sentences.
But, AI content detectors have their limits. A study at Cornell University showed they can be tricked easily. Theyโre good at spotting human texts but not so much with AI texts like GPT-3. This shows the constant fight between AI making and detecting content.
How AI Text Detection Works
AI text detection works by analyzing the linguistic patterns, syntax, and semantics of a given text to determine whether it was generated by a human or an artificial intelligence (AI) language model. This process involves the use of natural language processing (NLP) and machine learning algorithms to identify the characteristics of AI-generated text. AI detectors use various techniques, such as classifiers, embeddings, perplexity, and burstiness, to analyze the text and make a prediction about its origin.
Classifiers are machine learning models trained on labeled datasets of human-written and AI-generated text. These models learn to recognize patterns and features unique to each type of text, enabling them to classify new, unseen text accurately. Embeddings represent words or phrases as vectors in a high-dimensional space, allowing AI detectors to capture the meaning and context of the text. Perplexity measures the modelโs โsurpriseโ or โuncertaintyโ when encountering a new piece of text, while burstiness compares the variation of sentences to identify when a burst occurs.
By combining these techniques, AI detectors can accurately identify AI-generated text and distinguish it from human-written text. However, the accuracy of AI detectors can vary depending on the quality of the training data, the sophistication of the AI language model, and the type of text being analyzed.
Key Technologies Behind AI Text Detection
AI text detection uses advanced tech to find computer-made content. An AI detector, a tool specifically designed to analyze and assess the authenticity of written content, plays a crucial role in this process. The accuracy and functionality of these detectors heavily depend on the quality and type of training data used. Letโs look at the main parts that make this work.
Natural Language Processing (NLP)
NLP and semantic analysis are key to AI text detection. They let machines get and understand human language using an AI detector. By looking at text structure, grammar, and word use, NLP finds out if text is human or AI-made.
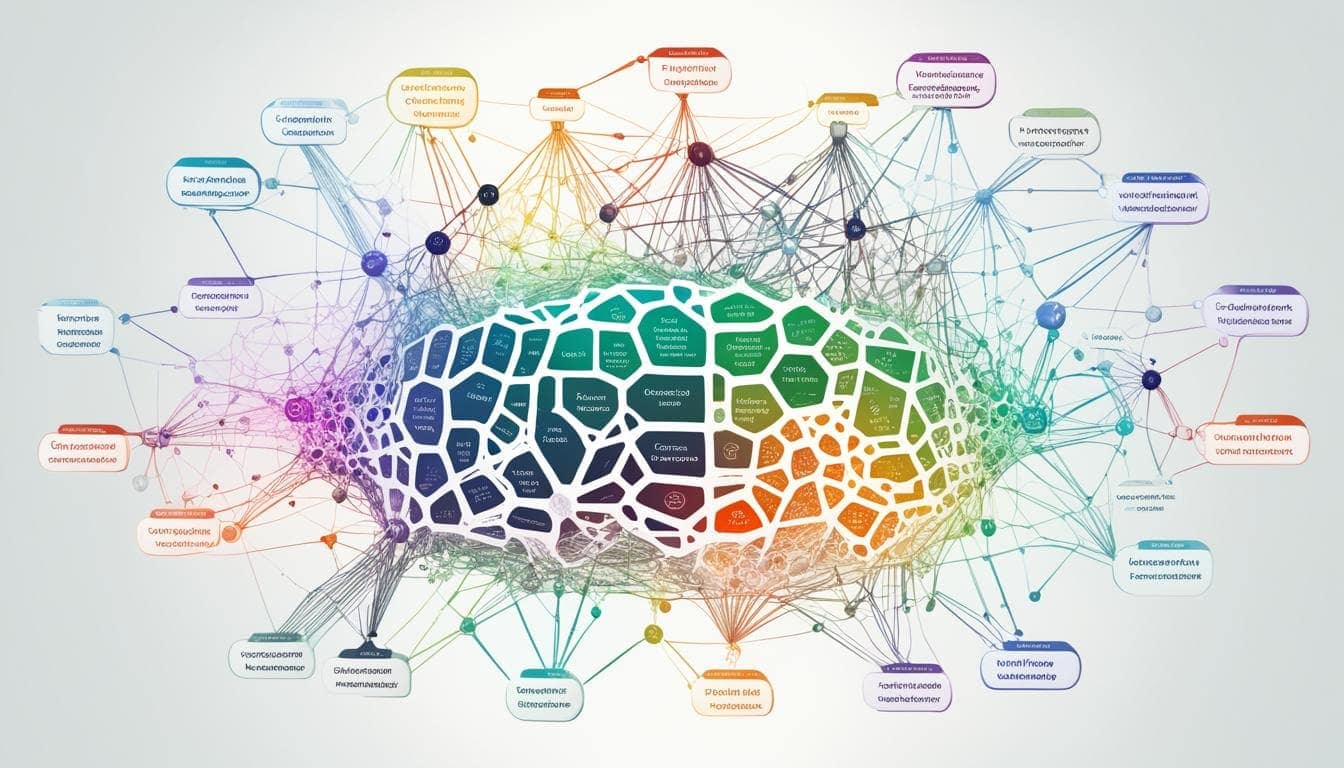
Machine Learning Models
Machine learning makes AI detectors better over time. These models learn from lots of human and AI texts, using pattern recognition to identify special traits in writing styles to better spot AI content, but ai detection tools have limitations and should not replace human judgment, especially in areas like SEO and fact-checking.
Deep Learning Techniques
Deep learning takes AI detection further. Neural networks look at complex text patterns. This helps them spot small writing differences, making it tough for AI content to go unnoticed.
AI algorithms use these techs to check text very accurately. They look at things like how rich the vocabulary is and how complex sentences are. Putting NLP, machine learning, and deep learning together makes strong tools for checking text and verifying content.
AI text detection uses advanced techniques to find machine-generated content. It explains the main methods, like linguistic analysis and text classification.
Classifiers
Text classification is key in AI detection. Supervised learning is used to train classifiers by looking at big datasets to find patterns in human and AI writing. Then, it puts new text into categories based on these patterns. This helps tell human from machine-written content.
Embeddings
Vector space models are the basis for word embeddings, which are crucial for AI text detection. They turn words into numbers for deeper analysis. By looking at these numbers, systems can spot small language differences. These differences might show if the text was written by a machine.
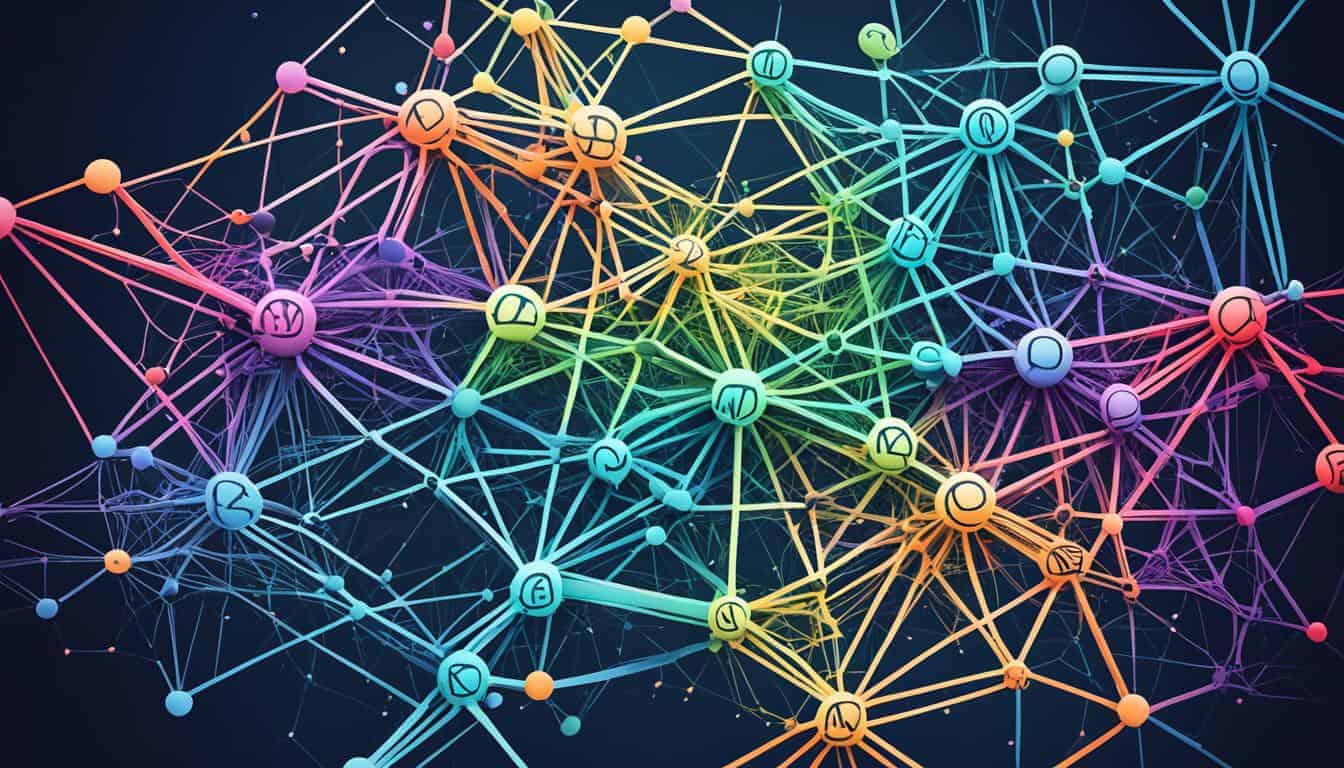
Perplexity
The perplexity measure is used in language modeling to show how surprised an AI model is by new text. Human writing usually gets higher scores because itโs less predictable than AI text. This helps systems spot possible machine-written text for closer look.
Burstiness
Burstiness looks at sentence structure and complexity through textual analysis. Human-written text has more variety in this area. AI tools check for these patterns to find signs of machine-generated text. Such text often lacks the flow of human writing.
Techniques for Identifying AI-Generated Content
Identifying AI-generated content requires a blend of sophisticated techniques that scrutinize various aspects of the text. Here are some of the most effective methods:
Natural Language Processing (NLP): NLP is a cornerstone of AI content detectors. It involves using algorithms to process and understand human language, enabling detectors to recognize subtle differences between human-written and AI-generated content. By analyzing text structure, grammar, and word usage, NLP helps in distinguishing the nuances of human writing from machine-generated text.
Machine Learning Models: These models are trained on extensive datasets comprising both human-written and AI-generated content. Through this training, they learn to identify patterns and anomalies unique to each type of writing. This continuous learning process enhances their ability to detect AI-generated content accurately.
Deep Learning Techniques: Advanced AI detectors employ deep learning techniques, such as neural networks, to boost accuracy. These techniques involve complex algorithms that can learn and improve over time, making it possible to recognize intricate patterns and anomalies in the text that simpler models might miss.
Cross-Referencing and Contextual Analysis: To enhance reliability, AI detectors often cross-reference content with existing databases and perform contextual analysis to understand the context in which the content appears. This method helps verify the accuracy of the content and detect any inconsistencies, ensuring a more thorough examination.
Detecting AI-generated content is crucial for maintaining content integrity in various fields, such as marketing, academia, and freelance writing. Specialized AI detection tools like Sapling, Crossplag, ZeroGPT, and Originality AI are effective, but human oversight is needed to verify the accuracy of the detection results.
How AI Detectors Analyze Text
AI detectors employ a multi-faceted approach to analyze text, examining various features to identify AI-generated content. Hereโs how they do it:
Syntax and Semantics: AI detectors scrutinize the syntax, semantics, and syntactic patterns of the text to identify patterns and anomalies. This involves examining sentence structure, grammar, punctuation, and the meaning of words and phrases. By understanding these elements, detectors can spot inconsistencies that may indicate AI-generated content.
Language Patterns: AI detectors look for language patterns typical of AI-generated content, such as repetitive language, keyword stuffing, and a lack of personal touch. These patterns often differ from the more varied and nuanced language used in human writing.
Tone and Style: The tone and style of the text are also analyzed to identify inconsistencies. AI-generated content may lack the unique voice and emotional depth found in human writing, making it easier for detectors to spot.
Context: AI detectors consider the context in which the content appears, including the author, the publication, and the purpose of the content. This contextual analysis helps in verifying the authenticity and relevance of the text.
Accuracy and Limitations of AI Detectors
AI detectors are key in telling human-written from AI-generated content. They say they can spot AI content over 90% of the time in tests. In my tests, they were right 7 out of 10 times with 100 articles.
Reliability of AI Detectors
The trustworthiness of an AI detector depends on many things. For example, a study found they work better with GPT 3.5 than GPT. This shows the challenge of keeping up with new AI models. Some tools, like ZeroGPT, say theyโre over 98% accurate. But, this might depend on the test conditions or the data used.
Common Challenges and Limitations
AI detector tools face many challenges in analyzing and assessing the authenticity of written content, including dealing with false negatives. AI detectors often wrongly flag human-written content as AI-made, especially in short texts. This can hurt the trust in content and cause unnecessary checks. They also donโt do well with languages or styles that are more creative or use many languages. As AI writing tools get better, AI detectors need to keep up to stay good at spotting AI content.
Ethical Considerations in AI Text Detection
The use of AI text detection tools raises several ethical considerations that must be addressed to ensure fair and responsible use. One of the primary concerns is the potential for algorithmic bias in the detection algorithms. If the training data used to develop the AI detector is biased, the tool may be more likely to flag certain types of content as AI-generated, even if they are not. This could lead to unfair treatment of certain individuals or groups, particularly those who may use non-standard language or writing styles.
Another ethical consideration is the potential for AI text detection to be used as a tool for censorship. If AI detectors are used to identify and flag AI-generated content, it could be used to suppress certain viewpoints or ideas. This could have serious implications for free speech and the exchange of ideas, as content that does not conform to certain standards or perspectives might be unfairly targeted.
Finally, there is the issue of transparency. AI text detection tools are often opaque, making it difficult to understand how they arrive at their conclusions. This lack of transparency can make it difficult to trust the results of the detection tool, and could lead to misunderstandings and misuses of the technology. Users need to know how decisions are made and what factors are considered in the detection process.
To address these ethical considerations, it is essential to develop AI text detection tools that are transparent, unbiased, and fair. This can be achieved by using diverse and representative training data, and by implementing mechanisms for human oversight and review. Ensuring that AI detectors are used responsibly will help maintain trust and integrity in the content they are designed to protect.
Real-World Case Studies of AI Text Detection
Several real-world case studies highlight the effectiveness of AI text detection tools across various fields. In academic settings, AI detectors have become invaluable for maintaining academic integrity. For instance, a study by the University of California, Berkeley, demonstrated that AI detectors could identify AI-generated content with a high degree of accuracy, significantly reducing instances of plagiarism in student assignments. This has helped educators ensure that students submit original work, fostering a culture of honesty and accountability.
In the media industry, AI detectors play a crucial role in identifying AI-generated content and combating misinformation. A notable example is a study conducted by the New York Times, which found that AI detectors were highly effective in identifying AI-generated fake news articles. By using these tools, media organizations can maintain the credibility of their content and protect their audiences from misleading information.
The business world also benefits from AI text detection tools. A study by the Harvard Business Review revealed that AI detectors could accurately identify AI-generated content, such as fake reviews and testimonials. This capability is essential for businesses that rely on customer feedback and reviews to build their reputation and trust with consumers. By ensuring the authenticity of online reviews, companies can make more informed decisions and maintain their credibility.
These case studies demonstrate the potential of AI text detection tools to identify AI-generated content and prevent its misuse. However, they also highlight the need for ongoing research and development to improve the accuracy and effectiveness of these tools, ensuring they can keep pace with the evolving capabilities of AI-generated content.
AI Detectors vs. Plagiarism Checkers
AI detectors and plagiarism checkers are both tools used to analyze text, but they serve different purposes and use different techniques. Plagiarism checkers are designed to detect instances of plagiarism by comparing a given text to a database of existing texts. These tools use algorithms and text comparison to identify similarities in language, syntax, and structure between the given text and the database of existing texts.
AI detectors, on the other hand, are designed to detect AI-generated text by analyzing the linguistic patterns, syntax, and semantics of the text. These tools use machine learning algorithms and NLP techniques to identify the characteristics of AI-generated text and distinguish it from human-written text.
While plagiarism checkers can detect instances of plagiarism, they are not designed to detect AI-generated text. AI detectors, on the other hand, are specifically designed to detect AI-generated text and can provide a more accurate assessment of the textโs origin.
Tools and Software for AI Text Detection
Several tools, software, and algorithmic tools are available to help detect AI-generated content. Here are some of the most popular ones:
Originality AI: Originality AI is a widely-used AI content detection tool that leverages natural language processing to identify AI-generated content. Itโs known for its accuracy and ease of use, making it a favorite among content creators and educators.
GPTZero: GPTZero is another popular AI content detection tool that boasts a high degree of accuracy. Itโs designed to detect AI-generated content by analyzing text patterns and anomalies, providing reliable results for users.
Copyleaks: Originally a plagiarism detection tool, Copyleaks has enhanced its platform to detect AI-generated text. It uses advanced algorithms to compare content against a vast database, ensuring the authenticity of the text.
Turnitin: Turnitin is a well-established name in the academic world, known for its powerful plagiarism detection capabilities. It has now integrated AI writing detection, making it a comprehensive tool for ensuring content originality.
Applications of AI Text Detection
AI text detection is used in many areas. It changes how we check content for realness and honesty. This tech is making a big impact in different fields.
Academic Integrity
In schools, AI helps keep things honest through plagiarism detection. Teachers use it to find plagiarism. They check student work against many papers to make sure itโs original.
Content Marketing
Content marketers use AI to check if their work is new. This keeps their brand looking good. It’s great for companies that make a lot of content.
Legal and Compliance Monitoring
Lawyers use AI to check documents are real. It spots fake documents or wrong info. This makes legal work more trustworthy.
Tools like Turnitin and Grammarly are key in these areas. They check for plagiarism and make sure content is real. As AI gets better, we’ll see more ways it helps keep content honest.
User Experience and Interface Design for AI Detectors
The user experience and user interface design of AI detectors are critical factors in their effectiveness and adoption. A well-designed interface can make it easy for users to understand the results of the detection tool and take appropriate action based on those results.
One key consideration in the design of AI detectors is the need for transparency. Users need to understand how the detection tool arrived at its conclusions and what factors it took into account. This can be achieved through the use of clear and concise language, detailed reports, and analytics. Providing users with insights into the detection process helps build trust and ensures that the tool is used correctly.
Another important consideration is simplicity. AI detectors can be complex tools, and users may not have the technical expertise to understand their inner workings. A simple and intuitive interface can make it easy for users to use the tool, even if they do not have a technical background. Features such as easy-to-navigate dashboards, clear visualizations, and straightforward instructions can enhance the user experience and make the tool more accessible.
Finally, customization is essential. Different users may have different needs and requirements, and the interface of the AI detector should be able to accommodate these differences. This can be achieved through customizable settings and preferences, allowing users to tailor the tool to their specific needs. Providing different modes and options can also help users get the most out of the AI detector, whether they are looking for a quick overview or a detailed analysis.
By focusing on transparency, simplicity, and customization, developers can create AI detectors that are user-friendly and effective, ensuring that these tools are widely adopted and used to their full potential.
Integration of AI Text Detection in Existing Systems
The integration of AI text detection tools into existing systems is a critical factor in their adoption and effectiveness. System integration plays a crucial role in ensuring that AI detectors can be seamlessly incorporated into various platforms, including content management systems, learning management systems, and customer relationship management systems.
One key consideration in the integration of AI detectors is the need for compatibility. AI detectors need to work seamlessly with existing systems and integrate with a wide range of software and hardware platforms. This can be achieved through the use of APIs and other integration tools, which allow different systems to communicate and share data effectively. Providing customizable interfaces and settings can also help ensure that the AI detector fits well within the existing workflow.
Another important consideration is scalability. AI detectors need to handle large volumes of data and scale to meet the needs of large and complex systems. This can be achieved through the use of cloud-based infrastructure, which offers flexibility and scalability. Distributed processing and other scalability features can also help ensure that the AI detector can process data efficiently, even as the volume of content grows.
Finally, security is paramount. AI detectors need to protect sensitive data and prevent unauthorized access and use. This can be achieved through the use of encryption and other security measures, ensuring that data is stored and transmitted securely. Secure authentication and authorization mechanisms can also help control access to the AI detector, ensuring that only authorized users can use the tool and access its results.
By focusing on compatibility, scalability, and security, developers can ensure that AI detectors are effectively integrated into existing systems, providing valuable insights and enhancing the overall workflow.
How to Create High-Quality AI-Generated Text
Creating high-quality AI-generated text requires a combination of advanced language models, high-quality training data, and careful tuning of the modelโs parameters. Here are some tips for creating high-quality AI-generated text:
Use advanced language models: Utilize state-of-the-art language models, such as transformer-based models, that are capable of generating coherent and context-specific text.
Use high-quality training data: Employ large, diverse, and high-quality datasets to train the language model. This will help the model learn to recognize patterns and relationships in language.
Tune the modelโs parameters: Carefully adjust the modelโs parameters, such as the learning rate, batch size, and number of epochs, to optimize its performance.
Use human evaluation: Incorporate human evaluators to assess the quality of the generated text and provide feedback to the model.
Use post-processing techniques: Apply post-processing techniques, such as spell-checking and grammar-checking, to refine the generated text and improve its quality.
By following these tips, you can create high-quality AI-generated text that is coherent, context-specific, and engaging. However, itโs important to note that AI-generated text is not a replacement for human writing, and itโs always best to use AI tools as a supplement to human writing rather than a replacement.
The Future of AI Text Detection
The future of AI text detection promises exciting advancements and improvements. Hereโs what we can expect:
Improved Machine Learning Models: Future developments will likely include improved machine learning models that can learn and adapt to new patterns and anomalies in AI-generated content. These models will become more sophisticated, enhancing their detection capabilities.
Increased Use of Deep Learning Techniques: The use of deep learning techniques, such as neural networks, will continue to grow. These techniques will improve accuracy and enable detectors to recognize subtle patterns in AI-generated content that are currently challenging to detect.
Integration with Human Review: Combining AI detectors with human review will ensure the quality and authenticity of content. Human reviewers can provide the nuanced understanding that AI might miss, creating a more robust detection system.
Development of New Tools and Software: We can expect the development of new tools and software that can detect AI-generated content with a high degree of accuracy. These innovations will keep pace with the evolving capabilities of AI writing tools, ensuring reliable detection.
By staying ahead of these advancements, we can continue to ensure the integrity and authenticity of content in an increasingly AI-driven world.
Conclusion
AI text detection is changing fast and has big implications. These tools are getting better, using NLP and machine learning. They can now spot AI-generated content with 80% accuracy. The significance of an AI detector lies in its ability to analyze and assess the authenticity of written content, ensuring content integrity and combating academic dishonesty.
How we make content is changing a lot. AI text can miss out on personal stories and feelings. It often uses the same phrases too much. This makes it hard for teachers and creators.
AI is changing many areas. OpenAI is a big leader, controlling 36% of the market. But, there are still problems. AI can mistake non-native English speakers and canโt always explain complex ideas well. As we go forward, weโll see more AI and human-made content. This will shape how we communicate and be creative.